DIGITAL SOLUTIONS
SMS-based phishing attacks
Luxembourg has been experiencing a substantial increase in SMS traffic, including malicious SMS-based attacks.
October 25, 2019
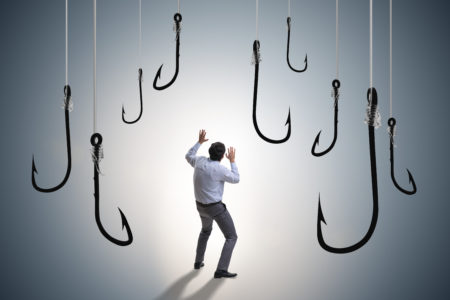
par Cu Nguyen
The SMS is coming back. Banking apps use it for authentication, commercial websites advertise by texting their customers, governments use text messaging to inform citizens, airlines use it to notify passengers about flight changes…
Moreover, with mobile virtual network operators (MVNO) leveraging Cloud computing and existing network operators, sending SMS has never been easier or cheaper.
However, this widespread use of SMS also benefits cyber-attackers, who are carrying more and more SMS-based attack campaigns for spamming, invading your privacy, threatening and phishing.
What phishing is
Phishing is an attack technique that consists in sending you – the rich and curious target audience – a message containing malicious URLs, luring you into following those harmful URLs. You are then asked to provide sensitive information, such as banking credentials or personal data. This technique proves to be effective as subscribers tend to trust SMS more than other means of communication.
At POST Luxembourg, we are committed to fighting SMS phishing through cutting-edge machine learning and real-time big-data technologies.
How machine learning automatically detects SMS phishing
The challenge we have to deal with is large-scale and real-time SMS phishing detection. It is interesting yet challenging at the same time. Some obstacles are:
– Short content
The short SMS only features a URL and a few words inviting you to open the link.
– The link cannot be inspected
Opening the link would invalidate it, just like with a reset password link.
– Inspection has to be 100% automatic
Manual inspection is neither allowed – due to privacy concerns – nor possible – due to the amount of data involved.
How to train the machine
From a machine learning perspective, we have to classify good URLs, like google.com, yourcompany.xx , etc. from bad URLs, such as apple-iforget.com orgooole.com.
This classification problem involves natural language processing. To solve it, we need to ask ourselves: What constitutes a bad URL? What are the tricks used by attackers? And so on. It turns out that there are over 30 features that define a bad URL. Below are some examples:
LIRE LA SUITE : https://ictexpertsluxembourg.lu/security-emm/fighting-sms-based-phishing-attacks/?utm_source=itnation%20phishing