TECH NEWS
Artificial Intelligence – The demystification of a myth
Ever since the phenomenon of ChatGPT, an AI system developed by openAI and launched in November 2022, the term artificial intelligence or AI is the subject of a new worldwide craze, effectively starting to revolutionize major aspects of our society already.
May 22, 2023
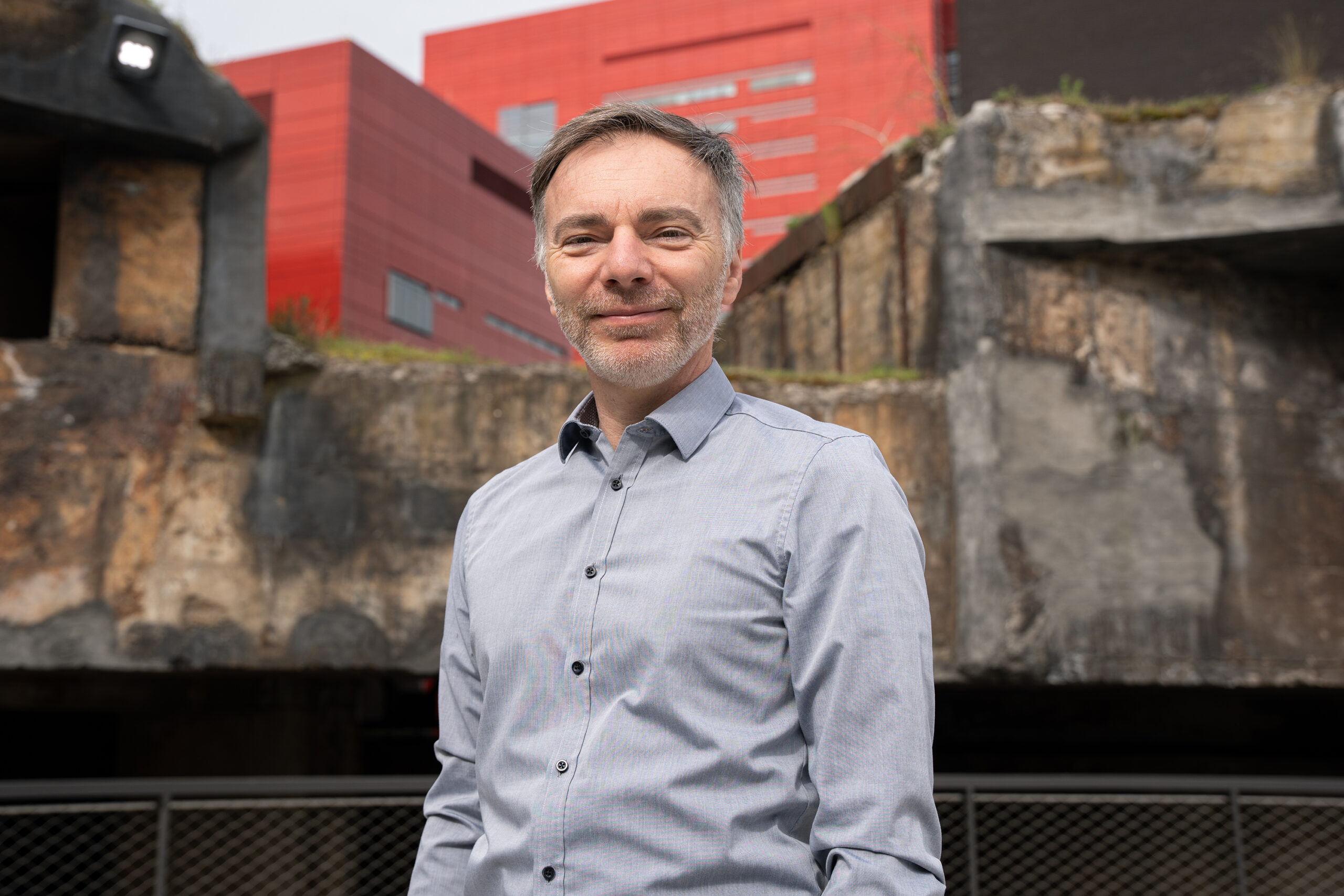
Ever since the phenomenon of ChatGPT, an AI system developed by openAI and launched in November 2022, the term artificial intelligence or AI is the subject of a new worldwide craze, effectively starting to revolutionize major aspects of our society already. Before this peak, AI as a field of science and research had unimpressive successes. Until now, AI, was a scientist’s dream made real on screen only in TV shows such as “Knight Rider” and popular movies like “Metropolis”, “Star Wars” and “The Terminator” to name just a few.
In 1956, the term “artificial intelligence” was coined by John McCarthy at Dartmouth College with the ambition to create machines that could simulate human intelligence and perform tasks that would normally require aspects of human intelligence such as reasoning, learning and problem-solving. A first popular example of an AI system was “Eliza” invented in 1966 at the Massachusetts Institute of Technology (MIT) by Joseph Weizenbaum. It was designed to engage in a conversation and to simulate an empathic psychotherapist. The approach was based on natural language processing and applying rules of grammar and syntax, rather than by understanding the meaning of the user’s input. These techniques allowed to generate follow-up questions by identifying keywords in the user’s input and responses.
In the late 1960s, Joshua Lederberg and Edward Feigenbaum created the first “Expert System” called “Dendral”. From there on, expert systems gained in popularity and aimed to replace human experts, especially in domains with a clear and rigorous set of rules, for example, law, chemistry, or customer support. These types of algorithms provided valuable recommendations based on a complex set of rules.
Humans gain intelligence by way of learning, which is a complex task. We learn from parents, teachers, peers, from what we read and watch on TV. In general, we learn from information that our brain receives all day long and that were captured by our senses. This huge amount of information that our brain receives consciously or subconsciously, is then processed, and eventually memorised for later retrieval. Our brain has fantastic optimisation capacities when it comes to the organisation of information in short-term or long-term memory. The challenge to our brain is to decide what information is useful and what is not, what is true and what is not.
An AI system faces similar challenges as it is fed with big amounts of data which is stored in its digital memory and kept handy to be processed later. Machines are used to find complex patterns (combinations of different features that occur again and again) in these large amounts of data. In this process called “machine learning”, the computer learns to pay attention to the details that matter.
In the field of machine learning, many methods use simple statistics and probability rules to figure out which features are most important for the task given the data. For example, machine learning methods are able to determine that the financial situation of a client is more important than their favourite colour if they want to get a loan. Since these methods are still limited to features (financial situation and favourite colour) that are predetermined by the programmer, the need to learn the features themselves was high. For example, when we want to classify flowers using images, the features that will be learned are combinations of the different pixels.
The new and high performing hardware made it possible to develop AI systems that could be trained on a large sample dataset. Once the system is trained and its performance is convincing, it can then be applied to new data samples from the same domain. For example: a neural network can be trained on a set of 10000 images of flowers for the task of classifying them into their predefined biological categories. After the training phase, new flowers that have not been seen before by the neural network, can be classified into their respective category if that category is known to the neural network. Whereas expert systems are based on the principles of logics and a given set of rules, machine learning algorithms learn the rules from available data using the principles of statistics and probabilities. Machine learning performs very well on previously challenging tasks, such as natural language processing, categorisation, and pattern recognition.
To illustrate the learning process, let us revisit the task of categorising flowers. Suppose we have two different types of flowers: tulips and roses. To train our AI system, we show it a small subset of available training data, for example, 10 randomly selected flowers from both categories. We let the AI system guess the category of each flower. For each flower, that has been wrongly classified, we “punish” the system based on how confidently it was while still being wrong. Repeating this step thousands of times, the AI system will learn to identify which general features, like the presence of thorns, are most important to differentiate flowers from both categories (tulips and roses). While humans are able to learn from small amounts of data, AI systems tend to require large amounts of data to learn patterns and features.
AI generally refers to a research field in computer science, whereas an AI system refers to a computer-based application of AI. An AI system can perform tasks that would typically require human intelligence such as object detection, classification, or speech recognition. AI systems can only become as good as the quality of the data on which they have been trained. If the data set is not diverse enough, meaning that it does not describe the task as a whole, the AI system will not be able to generalise its knowledge in that domain. Considering the previous example, a diverse dataset would incorporate many different individual flowers from both categories (tulips and roses). A general rule of thumb is the following:
“There is no data like more data” – Robert Mercer
Should humans be afraid of AI? While there is no need to fear AI as a whole, it is important to be aware of its potential risks and to work towards developing AI that is ethical, transparent, and accountable. This requires collaboration between policymakers, industry leaders, and researchers to ensure that AI is developed and deployed in a responsible and beneficial way. The answer to the question in this paragraph was completely generated by ChatGPT.
About the authors:
Dr Serge Linckels is the manager of the Digital Learning Hub, a structure that offers specialised IT trainings on site open to everyone without prerequisites. He is also deputy director of the Vocational Education and Training department at the Ministry of National Education, Children, and Youth. Serge has 22 years of experience as a teacher at the Lycée Guillaume Kroll and as an associate lecturer at the University of Luxembourg. He holds a degree in computer science and an engineering degree, as well as a PhD in Internet technologies and systems from the Hasso-Plattner-Institut. 30 scientific publications bear his name. He is the author of 3 books.
Ben Bausch coordinates the AI training branch at the Digital Learning Hub. He holds a MSc in AI from the Albert-Ludwigs University in Freiburg. His expertise is in unsupervised domain adaptation for semantic scene reconstruction in autonomous driving applications. His research interests are on using synthetic image data to train monocular depth estimation and semantic segmentation networks, while measuring their performance in real-world scenarios. He runs a YouTube channel “Minttube by Benimeni” on mathematical concepts, programming languages and machine learning algorithms in a simple and uncomplicated manner.